How life sciences manufacturers can implement Industry 4.0 tools
Artificial intelligence is skyrocketing – increasing the speed of work, raising productivity and providing new tools to help manufacturers scale their products safely and efficiently. There are few industries that stand to benefit from this revolution like the life sciences, where patient health and quality of life depends on the speedy delivery of breakthrough vaccines, therapies and cures.
As the world becomes more digital, companies are looking for ways to incorporate tools like artificial intelligence (AI), predictive analytics, and robotics into their daily processes, and the life sciences industry is following suit. In the fourth edition of the Horizons: Life Sciences report, data collected from more than 500 industry professionals reveals that most companies are budgeting for AI, yet remain behind in fully integrating Industry 4.0 technologies.
We are joined on this episode by CRBers Ryan Thompson, Senior Specialist of Industry 4.0; Niranjan Kulkarni, PhD, Senior Director of Consulting; and Peter Walters, Fellow, Advanced Therapies. They address the risks of adopting Industry 4.0 technologies, how those tools can be useful in producing treatments efficiently, and what tools and strategies are worth applying today.
Here’s a look at the episode:
02:19 – Our experts discuss generative AI, touching on its applications to the life science manufacturing process and potential to create personalized treatments for patients.
10:24 – Ryan explains that manufacturers must work in tandem with technology and provide it with high-quality data to receive optimal results.
15:29 – The team shares which tools are worth the investment and how instilling a digital-first culture will benefit companies.
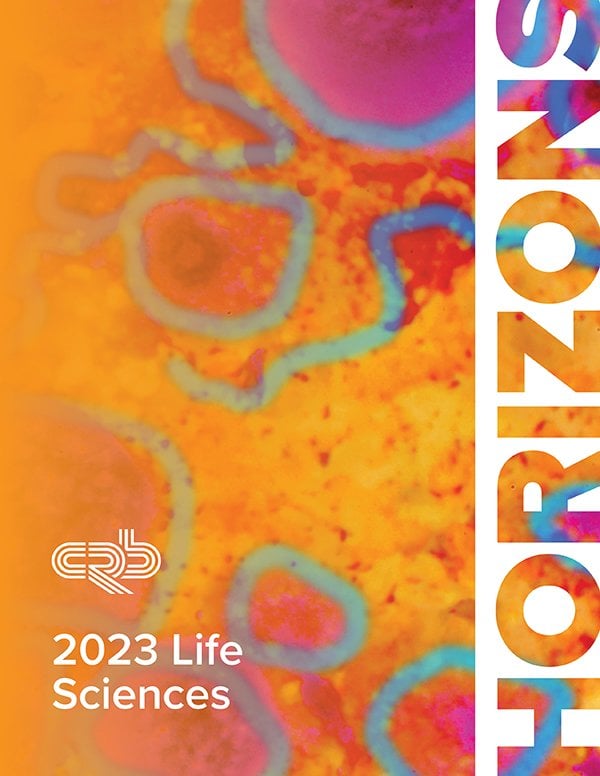
For a deeper dive into the industry’s embrace of Industry 4.0, download your copy of the 2023 Horizons: Life Sciences report:
Transcript of Episode 4: How life sciences manufacturers can implement Industry 4.0 tools
Ashley Martins: Welcome back to the CRB Horizons Podcast! We’re bringing you the trends and data collected from our survey of hundreds of industry leaders in the life sciences and food and beverage industries. Join me, Ashley Martins, as we dive into the 2023 Horizons: Life Sciences report with the subject matter experts who wrote it.
Ryan Thompson: My name is Ryan Thompson, Senior Specialist, Industry 4.0.
Niranjan Kulkarni: Niranjan Kulkarni. I’m the Senior Director for Consulting.
Peter Walters: I am Peter Walters. I’m a Fellow of Advanced Therapies here at CRB.
Ashley Martins: The 2023 Horizon’s: Life Sciences report captures data from more than 500 industry leaders working at small to large companies in Europe and North America and one theme stood out. We are eager to jump into digitalization.
The world is getting digital and fast. The life sciences industry in particular is adopting Industry 4.0, also known as the fourth Industrial Revolution. Digital technologies aid in improving manufacturing operations through tools like predictive analytics, robotics, automation, artificial intelligence and machine learning. Though the life sciences industry is behind others in the full adoption of digitalization tools, our findings reveal that most companies in this space have the budgets to begin supporting these efforts.
High quality contextualized data and a robust strategy unlock the potential to implement advanced analytics, artificial intelligence and machine learning at scale. Even with the advancement of these exciting new technologies, life science manufacturers are still hesitant to fully invest in Industry 4.0 in their facilities. In today’s episode, Ryan, Niranjan and Peter talk through these challenges and risks the industry faces to adopt industry 4.0 technologies. These include needing a better way to quickly deploy medicine and treatment to patients, keeping patients safe by ensuring quality in the treatment manufacturing process, and adhering to local regulations.
The first tool we discuss is generative AI. How can it be useful for life science manufacturers, especially when it comes to personalizing treatments for people and producing medications quickly?
Ryan Thompson: Artificial intelligence is a big topic. I actually think if you look at a lot of market research, it’s the first year, you know, 2022 into 2023 where CEOs are mentioning this in their earnings reports, what they’re doing with AI. You saw it from like a pop culture point of view with ChatGPT, and that ChatGPT is an example of generative AI, which is artificial intelligence that’s creating content as opposed to artificial intelligence, that’s looking at data and giving insights.
So the biggest use case that we see, and this is in the Horizons report as well with generative AI, is in like drug development, so designing new molecules. I think that’s where most companies are investing actively right now. But I also think there’s a lot of opportunity outside of that, specifically in the manufacturing space. How are we going to design new processes not from like a process tech point of view, but like from like an organizational intelligence point of view? Does it make sense to have a person do task A-B-C or can we design our whole operation differently? You know, taking a shift-based smart manufacturing approach as opposed to, I’m going to dedicate this person to this piece of equipment.
So from, like, a personalized medicine point of view, you can’t have these people dedicated to like a bench and you’ve got like, process variability to consider. So you might have a bottleneck on one piece of equipment and an operator standing by idle. AI should be able to better generate solutions to run our businesses. I think we’re kind of behind on that. When you look at what AI has done to other industries like banking and retail, there’s a huge shift. Manufacturing is way behind and life sciences are way behind as well compared to manufacturers in general. I think that’s from a regulatory perspective, it’s a lot more difficult to implement solutions in a life sciences environment than it is in other environments, but there’s a lot of opportunity. And like what this report really shows is that our clients and the industry is investing significantly and they’re ready to adopt this change. I think they do need some more guidance from industry and that could be organizations like ISPE, but mostly regulators as well FDA or European Medicines Agency.
Niranjan Kulkarni: Yeah, I mean, there is, as the report also shows, you know, there is a lot of interest and actual applications upstream in the drug development. But as you rightly said, it is trickling its way down into manufacturing. We have seen some use cases where people are now moving away from a reaction like maintenance is going more towards condition-based, more predictive, truly. Where AI can figure out, what’s the perfect storm? When do you need your maintenance crew to be ready? So I think there are applications that we are seeing. Yes, they are still in the making, but I think that has tremendous potential in commercial manufacturing as well.
Peter Walters: I think not even just the building facilities and the equipment side of it, but even pushing into the process. We’re even seeing some situations with, especially when talking about personalized medicine, where you have this very complex sort of manufacturing ecosystem where you’re starting material is varying every single batch, trying to figure out what your critical parameters are and how to put ranges on those is very difficult. And we’ve even seen some companies that are trying to leverage AI systems to help inform them real time about how to perform their process to get the best possible outcome. And in terms of a quality product, you might get the best quality product out of that because you’re doing huge number of computations in a way that humans just can’t.
Ryan Thompson: I mean, I think you raise a really good point there. Humans just aren’t good at looking at all this type of data in real time and providing solutions Whereas computers, they sit by idly, but they’re able to like grasp things that you would never think would be related and just ingest so much data and come up with these insights. Like, you can have a team of scientists looking at that.
But I think something that’s critical is making sure that we’ve got data strategies in place to make sure that your whole business is structured to ensure that data is being able to be consumed by these AI or advanced analytics programs. Because so much time right now, even if you look at a data scientist’s time, they spend three quarters of their time cleaning and preparing data to be ingested into these systems, and that’s really not going to scale either.
Peter Walters: Yeah.
Niranjan Kulkarni: But I don’t think there’s going to be a Terminator coming out of this, I’m sure. So humans are still going to be very critical in interpreting that data. Yes, the AI can find the patterns. But how do you interpret or how do you apply that?
Ryan Thompson: Yeah and how do you prove it to regulators, too, like Peter mentioned?
Niranjan Kulkarni: Absolutely. And I think we are very focused on, yes, manufacturing and facilities for the right cause, but I think it extends into supply chain as well. Right? Well, especially for personalized medicines, if it is one batch, one patient, knowing that it’s going to the right patient is critical. How do we adjust our parameters? How do we find the optimized routes? Which, many times, the retail industry has done a decent job at that, right? Amazon made sure that there are as minimum left turns as possible because right turn is free. So having those kind of insights into logistics, AI can truly help fasten the overall supply chain, not just manufacturing I feel.
Ryan Thompson: I mean, I think on the personalized medicine side, life sciences companies have to become logistics companies like a little mini-Amazon because that problem is so difficult to solve and it’s not one that they’ve been thinking of. Imagine from a health care provider’s point of view, how do you know that the manufacturing facility has the capacity to make what you need to make if you go in to see your doctor, get your patient material drawn? How do you know your therapy is going to make it back to you on time? How can the hospital rely on the manufacturer if these things aren’t completely integrated and tight knit? And like we can talk about, you know, building factories bigger than they need to be, so we know that the capacity is available all the time. But that’s just going to increase costs drastically for products that are already really expensive, where we need to as an industry drive towards reducing those really by 90% or something like crazy to get them to be commercially viable products.
So I think a lot of people have heard the phrase data is the new oil. It’s something that’s a little bit buzzy, but it’s really about getting extra value from the information that you’re collecting already. So you can think about if, you know, oil companies make a lot of money by extracting oil from the ground. But what are you going to do with data to get value out of it? It’s of no value to anyone if you’re collecting information, whether that’s from your manufacturing operations or any other business system that you might have, it just sits on a hard drive somewhere and you don’t do anything with it that’s of no value to anyone. It’s actually a cost because now your cost, you’ve got to store it, you have to maintain that storage, and especially in life sciences with all the regulation about keeping it with you. And if you’re not going to do anything with it, then what’s the value? Companies today, if you look at it like a lot of people say, like Tesla is a data company that makes cars, so their products continue to get better as you use them because it’s collecting a whole bunch of information for how people drive their cars and making that user experience better. I think that’s what part of digital transformation is unlocking new value streams based on the data that’s being collected and that you’re getting anyway. I think something that’s really exciting at least on like the personalized medicine side, you can see what the patient results are with the therapy that they received. And I mean, there’s privacy concerns that we’ll have to deal with as an industry with that, but I think that can be really powerful.
Ashley Martins: Tailoring medicine directly to the patient while delivering quick, reliable and consistent turnaround times at a lower cost would be a huge breakthrough in the life sciences industry. However, it comes with another consideration. We are dealing with people’s lives. If we hand the responsibility over to AI or technology, what happens if the tech gets it wrong and patients experience detrimental side effects? What risks are life science manufacturers willing to take?
Peter Walters: They have a business to run, and their business is, of course, at risk when they make major changes. But they’re also putting the patient population at risk when they’re making changes that could significantly interrupt the supply chain of therapies to the marketplace. How scary do you think it is for companies to be taking basically roles that have historically been performed by people and handing them over to an AI system to now be managing in a way that maybe or do you feel like there’s just like a general lack of understanding as to how that works and how that’s going to impact? Is it going to be making mistakes like things like that?
Ryan Thompson: So I don’t think that companies are ready to take that step yet where AI is completely managing everything. Where I’ve seen companies be successful is AI makes recommendations and then a person would review those recommendations and see if they’re appropriate and gauge the risk. So AI is kind of looking at everything and saying, “Hey, this doesn’t look right to me. What do you think about it?”
So it’s not directly making the GMP decisions and the person is, of course, but that can become another problem where if AI gets good and 90% of the recommendations are right, people can get complacent in that, too, right? So you have to look at that operational risk and what that means. But I think right now companies aren’t ready to take that full, “Hey, AI says this.” And that’s really how they’re structuring their validations and their change managements as well.
Niranjan Kulkarni: But I also think the AI is learning, right? It’s a machine learning. What if it is learning the wrong thing?
Ryan Thompson: And so that’s a really good question because it’s going to learn how you operate.
Niranjan Kulkarni: So if Tesla’s going to learn my driving style, it’s just learning the wrong thing. And if it continues to learn, it’s actually not learning. It is it is learning, but it’s learning the wrong way, the wrong thing. And if that is going to be implemented, it’s going to be a disaster. So who’s going to check if it is learning the right way or not?
Ryan Thompson It’s a really good point. I mean, the more data you collect, the better it’s going to be. But I think what’s tricky for manufacturers and life sciences, in particular, is that everybody considers their data proprietary. So, I mean, when we talk about data that is the new oil, keeping that to yourself means that’s where, you know, I’m going to make the most money; my business is going to be the most successful. Where AI gets really, really good. And we talked a little bit about predictive maintenance. Nobody cares what the performance of a motor is for vibration and temperature. It’s going to fail. You know what the profile looks like. Nobody considers that a proprietary thing. But how you operate a manufacturing facility, people do and should consider that proprietary, whereas if we take a step back as an industry, it would be better if everybody shared all of their data and then we could, you know, determine which companies are successful, what is success, what is right. But nobody’s going to do that. I think that’s not the way the world works, but to determine what’s the right thing, you have to really look at what KPIs you’re looking for, what actions turn into business results and having it learn that. It would still identify what’s good for you, even if that’s the wrong thing.
Niranjan Kulkarni: It doesn’t matter if it’s AI learning or a person doing it. These doses are administered to the patient when they are literally, you know, on their last string. Right? And if string breaks, do we know it is because the medicine did not work. So how do we know that there was a quality issue in the medicine?
Peter Walters: So I mean, the way the way that you would typically be trying to frame that is you have a set of procedures. And as you’re doing these procedures, there’s typical ranges you have to be staying within. The idea being that if you’ve done everything with these steps and the testing that you’ve been doing falls within its normal ranges, as much as you can guess, that drug is going to be consistent with the name, the label, the claims, the clinical data, the performance of it. But to Ryan’s point earlier, not every product is going to work 100% of the time. A lot of times that is just unrealistic. When we talk about medicine and the complexity that is the human immune system and whatnot, you’ll have a percentage chance that it will work a percentage amount of time or a percentage clearance or a percentage cure, or it will address the symptoms some amount. And that’s going to change person by person by person anyway. And ultimately, it will come down to the interaction between the patient and the medication and how well those two function together. That might have nothing to do with the medication itself.
Ashley Martins: There is a lot of skepticism around AI. What are some strategies companies can take to be innovative? And what, if any, new technology is worth investing in?
Ryan Thomspon: So I think as in any industry, especially and I’m in a pretty big niche with Industry 4.0, it becomes like an echo chamber. And people hear a lot of buzz words, like you’ll hear people like me going and say, “You have to do this, this and this.” And they just don’t see the business case around it. And they’re like, “Well, if this is so great if you’re going to get this tax return on investment right away, wouldn’t everybody just be doing this?” I think there’s a lot of people that have been, you know, sold one thing and delivered something else. So they have that kind of natural cynicism, so you have to implement these things and really learn. And if you’re not being an innovative company, if you’re going to just throw off these technologies, I think it’s a mistake, but you’re certainly welcome to do that. That’s everybody’s business perspective. If they don’t believe in the hype, then they don’t believe in the hype.
But I think what we really need to do as an industry is deliver results. And if we can show we did this and here were the results, I think that’s a lot more powerful than talking about high level, “Oh, you could do this, you could do this, you could do this.” So, you know, I like to look at every client that I’ve ever worked with, every factory I’ve ever been in, there’s low hanging fruit to do these types of digital transformations or digital proof of concept. I think it’s a matter of, like I said, just delivering results quickly and it will build confidence and it creates a snowball effect. I think it’s also like a cultural thing where you really need this led from the leadership level of an organization that says, “We’re going to go down this path. We believe culturally it’s the right thing to do. We’re going to make mistakes along the way and we’re going to fail fast and fix them.”
And again, in life sciences, it becomes a bit more difficult because when you’re making GMP decisions with the data or using artificial intelligence or data for any GMP purposes, it becomes like a validation headache. Whereas in like in other industries, you’re able to take a more agile project methodology, agile project management methodology, do sprints, iterate, fail fast, quick fix, and you start getting results like your time to value is a lot faster. I think in life sciences you really need your, you know, change management and organizational procedures set up in a way that enables that agility as well.
Niranjan Kulkarni: Yeah, to add to that, I think it’s not just the change management and the cultural aspect, but you hit on the point I think that’s important is selecting the right project is going to be important. We don’t want to undertake a big project that take a long time, you know, takes a lot more money than you want to spend and then it doesn’t give the results at the end. So selecting the right project, implementing fast. And I like what you said is fail fast as well, so you can learn from it, get up and hopefully it’s a small project that does not impact the bottom line.
Ryan Thomspon: Sometimes it’s tricky too, because a lot of the investments that you need to make are infrastructure style investments. So it’s hard to develop like a return on investment for a proof-of-concept project because you end up spending three quarters of your project budget on infrastructure, which can be expensive. So that can be a challenge as well. But when you look at a lot of software as a service offerings or cloud-based offerings, I think that’s a nice bridge into those things where you can, you know, you turn it on for a proof-of-concept project and if it’s not producing value, you shut it off and that’s that. You haven’t spent any capital. And if it’s successful, it’s really easy to justify additional investment.
Niranjan Kulkarni: So do you know what technologies could be considered hype versus you have to do something?
Ryan Thomspon: So I think with life sciences specifically, electronic batch records and released by exception is you have to do something especially on like the personalized medicine point of view that you just can’t scale without that. I do think in general having a data strategy is a really good investment that you can make. So how do you deal with data from its conception through completion, like when it is originated, to when it’s deleted. So that’s going to be a really complicated process because if you have records of something, whether or not you’re using it, you’re responsible for the maintenance if an FDA comes in and audits you, you have to be able to provide this information so it can be expensive to maintain data that you don’t need. So having a data governance strategy is a really important and essential piece, and that will enable other technologies like artificial intelligence and machine learning.
Some things like digital twins are really exciting, but those can be significant investments depending on the amount of fidelity you want to put into those models. So to me, that’s not a must have that’s kind of a nice to have type of technology and really investing in like the cultural piece of this. We’re going to be a digital first company. This is the way we’re going to do things. We’re going to make our make sure our whole business is connected, make sure our manufacturing floor is connected to our business systems. And just that type of change can provide a lot of value and really enable people to do their jobs better, enable them to make changes to whether that’s pieces of software or procedures. I think it also makes your company a more exciting place to work. If you feel like your whole staff is empowered to use all the data that’s collected to make their part of the company perform better, I think that’s going to produce incredible results. And I think the cultural side is a big place to invest as well.
Ashley Martins: Industry 4.0 and its tools are not going anywhere, but people and processes are just as important as the technology. Having a leadership team in place that facilitates a digital-first manufacturing mindset enables the success of these technologies and empowers everyone in the organization to make Industry 4.0 a reality. With the right team, creating a data strategy, leveraging it to solve specific use cases, and putting it into practice will make a facility more agile and better equipped to produce therapies efficiently.
Want more information about Industry 4.0 and how it’s affecting the life sciences industry? Download your copy of the free Horizons: Life Sciences report by visiting crbgroup.com/horizons-reports. We will share those links in the show notes. Subscribe to the show so you can be notified when our new episode is released next month. Thanks for listening!